
SMART MOBILITY FRAMEWORK: TRI-POP
Advances in technology (information, connectivity, automation) are affecting all components of urban mobility. These trends facilitate novel mobility solutions for passenger and freight transport that are on-demand, shared, and integrated. Next generation mobility management systems and services should leverage these advances in technology and employ online analytics to achieve three key features: (1) prediction, (2) optimization and (3) personalization. Prediction is key to adapting transportation systems to changing conditions in the real-world and facilitating proactive decision-making. Transportation system predictions should utilize real-time data and explicitly consider demand and supply-side characteristics and their interactions. Optimization is critical in order to achieve system- and operator-level objectives while ensuring fairness, e.g., minimizing network-wide travel times, maximizing revenue and/or consumer surplus. Personalization is key to maximizing users’ benefits through better understanding of their individual preferences. The key challenge is how should these features be combined in real-time to design ‘smart’ mobility solutions?
The ITS lab has developed a generic platform for Smart mobility termed Tri-POP. The platform consists of a user experience module interacting with a personalized user-optimization module (user level) and a prediction-based system optimization module (system level). Users make trip requests or queries to the user experience. Each time a user makes a query, the personalized user-level optimization runs to determine the offered service options subject to the system-level optimal policy in effect. The user-level objectives may be consumer surplus, time and energy savings, etc. A key element of the user-level optimization is a user-specific behavioural model based on stored historical user-specific data (user’s preferences are collected each time a choice is made on the user experience) and contextual data. The system-level optimal policy is determined in real-time periodically by the prediction-based system optimization with the objective of attaining system-level objectives such as minimum travel time and energy consumption, maximum social welfare, etc.
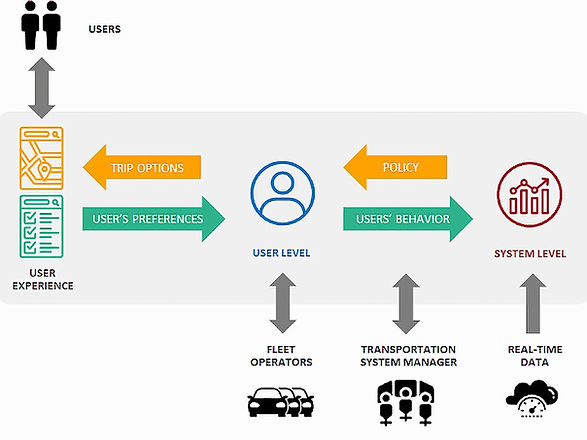